4.6: microRNA (miRNA) Gene Signatures
- Page ID
- 40932
One example of functional genomic regions subject to high levels of conservation are sequences encoding microRNAs (miRNAs). miRNAs are RNA molecules that bind to complementary sequences in the 3’ untranslated region of targeted mRNA molecules, causing gene silencing. How do we find evolutionary signatures for miRNA genes and their targets, and can we use these to gain new insights on their biological functions? We will see that this is a challenging task, as miRNAs leave a highly conserved but very subtle evolutionary signal.
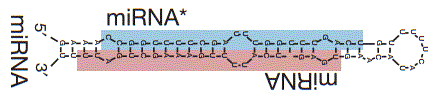
Computational Challenge
Predicting the location of miRNA genes and their targets is a computationally challenging problem. We can look for “hairpin” regions, where we find nucleotide sequences that are complementary to each other and predict a hairpin structure. But out of 760,355 miRNA–like hairpins found in the cell, only 60–100 were true miRNAs. So to make any test that will give us regions statistically likely to be miRNAs, we need a test with 99.99% specificity.
Figure 4.25 is an example of the conservation pattern for miRNA genes. You can see the two hairpin structures conserved in the red and blue regions, with a region of low conservation in the middle. This pattern is characteristic of miRNAs.

By analyzing evolutionary and structural features specific to miRNA, we can use combinations of these features to pick out regions of miRNAs with >4,500-fold enrichment compared to random hairpins. The following are examples of features that help pick out miRNAs:
- miRNAs bind to highly conserved target motifs in the 3’ UTR
- miRNAs can be found in introns of known genes

Figure 4.26: Novel miRNA in intron© source unknown. All rights reserved. This content is excluded from our Creative Commons license. For more information, see http://ocw.mit.edu/help/faq-fair-use/.
- miRNAs have a preference for the positive strand of DNA and for transcription factors
- miRNAs are typically not found in exonic and repetitive elements of the genome (counter-example in Figure 4.29).
- Novel miRNAs may cluster with known miRNAs, especially if they are in the same family or have a common origin

These features of miRNA-coding regions can be grouped into structural families, enabling classifiers to be built based on known RNAs in each family. Energy considerations for RNA structure can be used to support this classification into families. Within each family, orthologous conservation(genes in different species for same function with common ancestral gene) and paralogous conservation (duplicated genes within same species that evolved to serve different functions) occurs.
Evolutionary
- Correlation with conservation profile
- MFE of the consensus fold
- Structure conservation index
Structural
- Hairpin stability (MFE z-score)
- Number of asymmetric loops
- Number of symmetric loops
We can combine several features into one test by using a decision tree, as illustrated in Figure 4.28. At each node of the tree, a test is applied which determines which branch will be followed next. The tree is traversed starting from the root until a terminal node is reached, at which point the tree will output a classification. A decision tree can be trained using a body of classified genome subsequences, after which it can be used to predict whether new subsequences are miRNAs or not. In addition, many decision trees can be combined into a “random forest,” where several decision trees are trained. When a new nucleotide sequence needs to be classified, each tree votes on whether or not it is an miRNA, and then the votes are aggregated to determine the final classification.
Applying this technique to the fly genome showed 101 hairpins above the 0.95 cutoff, rediscovering 60 of 74 of known miRNAs, predicting 24 novel miRNAs that were experimentally validated, and finding an additional 17 candidates that showed evidence of diverse function.
Unusual miRNA Genes
The following four “surprises” were found when looking at specific miRNA genes:
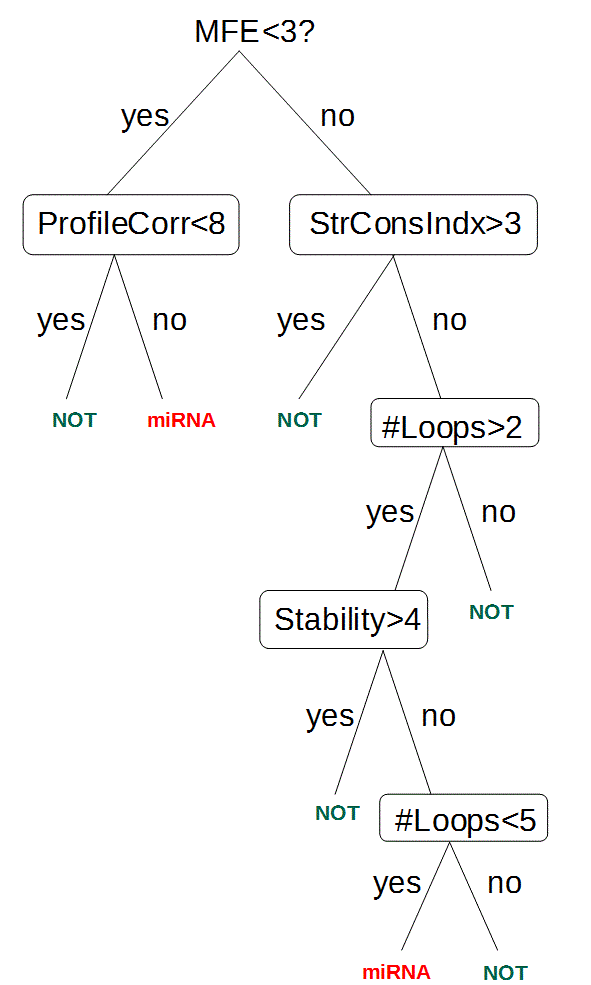
Surprise 1 Both strands might be expressed and functional. For instance, in the miR–iab–4 gene, expression of the sense and antisense strands are seen in distinct embryonic domains. Both strands score > 0.95 for miRNA prediction.
Surprise 2 Some miRNAs might have multiple 5’ ends for a single miRNA arm, giving evidence for an imprecise start site. This could give rise to multiple mature products, each potentially with its own functional targets.
Surprise 3 High scoring miRNA* regions (the star arm is complementary to the actual miRNA sequence) are very highly expressed, giving rise to regions of the genome that are both highly expressed and contain functional elements.
Surprise 4 Both miR–10 and miR–10* have been shown to be very important Hox regulators, leading to the prediction that miRNAs could be “master Hox regulators”. Pages 10 and 11 of the first set of lecture 5 slides show the importance of miRNAs that form a network of regulation for different Hox genes.
Example: Re-examining ’dubious’ protein-coding genes
Two genes, CG31044 and CG33311 were independently rejected because their conservation patterns did not match those characteristic of a protein evolutionary signatures (see Section 4.5). They were identified as precursor miRNA based on genomic properties and high expression levels (Lin et al.). This is a rare example of miRNA being found in previously exonic sequences and illustrates the challenge of identifying miRNA evolutionary signatures.
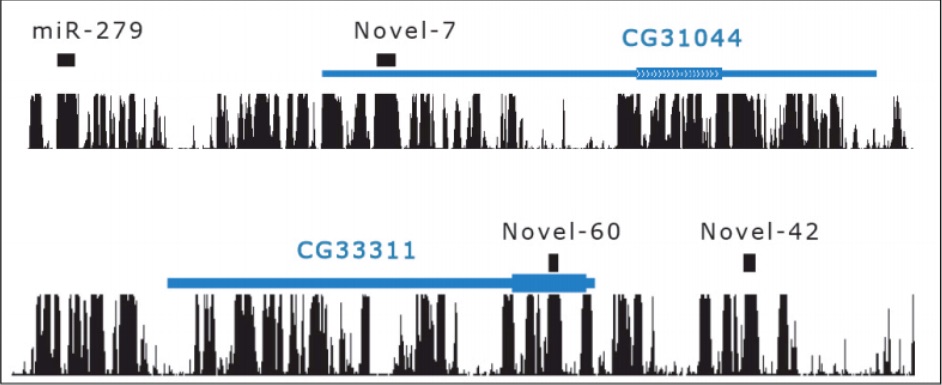